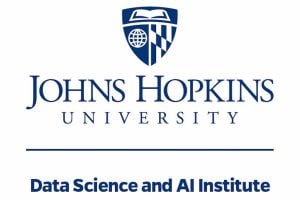
In 2024, the Johns Hopkins Data Science and AI Institute awarded two Johns Hopkins faculty members the first Trusted Dataset Awards. The awards provide selected researchers the opportunity to further the Data Science and AI Institute’s mission to establish Johns Hopkins as a premier source of trusted datasets for research, scholarship, and translation.
“A principal goal of the Trusted Datasets Awards was to begin building a body of tools and datasets broadly useful for AI researchers with a particular focus on the data,” says Jim Bellingham, the Data Science and AI Institute’s research director for safety and assurance. “We are excited by the results of this first round of research, the first of what we hope to be many contributions to safer, more trustworthy AI.”
Mathias Unberath, a John C. Malone Associate Professor of Computer Science, received one of the awards to support his project, “A Trusted Living Dataset and Compute Infrastructure for Ambient Intelligence in JHM Operating Rooms.” Through this work, Unberath and collaborators in the Johns Hopkins Whiting School of Engineering, Johns Hopkins School of Medicine, the Applied Physics Laboratory, and the Armstrong Institute for Patient Safety and Quality, have created a trusted living dataset and developed sophisticated algorithms to advance ambient intelligence within the Johns Hopkins community.
The research team generated more than 50 videos that capture simulated operating room (OR) workflows, including OR staff reenacting surgical procedures. The team is developing a suite of tools and algorithms that support the detection of humans and objects in the OR. These toolsets, videos, and annotations comprise a dataset and infrastructure that can advance research in ambient AI for surgical environments. In doing so, Unberath’s team developed a new protocol for annotating surgical videos, which addressed limitations in previous related work. The protocol highlights the need for prespecified annotation vocabulary and dictionary, quality assurance, and control measures.
Additionally, Unberath and his team are working with Information Technology at Johns Hopkins to establish a multi-tiered storage and compute access framework that is HIPAA-compliant to protect sensitive patient health information. The goal is to make the dataset securely accessible through Johns Hopkins platforms to enable compliant and efficient model development and training.
Together, the videos, annotations, and toolsets have allowed Unberath and his team to establish and refine a data collection protocol, adding that these resources comprise a one-of-a-kind trusted dataset and infrastructure poised to significantly advance research in ambient AI for surgical environments.
“The Trusted Dataset Award allowed us to advance our work on ambient AI for operating room analytics, building our platforms for the secure handling of OR video data captured from both room-level and egocentric camera video feeds, as well as algorithms that handle de-identification among other processing steps,” says Unberath. “We will continue this line of work beyond the Trusted Dataset performance period, further enhancing and expanding the platform and building algorithms for automated data analytics to enhance OR efficiency.”
The second Trusted Dataset Award was presented to Swaroop Vedula, an associate research professor in the Malone Center for Engineering in Healthcare, for his work, “Datasets to Enable Trusted and Generalizable Artificial Intelligence for High-Stakes Assessment of Surgeons and Autonomous Feedback for Surgeons.” With support from the award, Vedula and his collaborators developed prototypes of resources that allow surgeons and engineers to create datasets for trustworthy AI for surgery.
Vedula shares that while using AI for surgery is predicted to rapidly expand and have a significant impact on the field, the current paradigm is to create large datasets within silos defined by investigators or institutions. “These data silos are necessary to protect patient privacy and confidentiality, but they stifle innovation using large datasets needed to train AI models. Our work creates the building blocks that make the silos transparent while protecting patient privacy and confidentiality. This transparency enables investigators to use all relevant data to develop AI models, which would otherwise not be possible,” explains Vedula.
The team established protocols for creating and sharing trusted annotations of surgical videos, created annotations for a public video dataset focused on cataract surgery, and developed a metadata model for datasets used in AI for surgery. Vedula is now leading the creation of a multi-institutional, multidisciplinary collaboration to complete this work and build a lasting community to sustain the effort and to assure its impact on AI innovation for surgery.
“Rapid advances in AI and sensing technologies promise to make surgery more safe, effective, and personalized to each patient. We must know what data exists so we can build the best AI using all available data,” says Vedula. “Our work is important because it allows us to know what data is available to develop AI models and to make the data interoperable. It also allows us to accelerate innovation, trusted evaluation, and translation of AI for surgery.”
Vedula will present at the Sip & Solve: AI Lightning Talks with a Twist event on May 6, hosted by the Johns Hopkins Data Science and AI Institute in partnership with the Applied Physics Laboratory.
Learn more about the Data Science and AI Institute Trusted Dataset Awards.