The Power and Promise of Data Science and AI
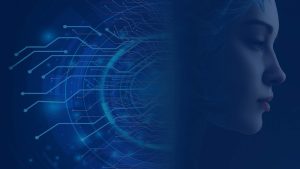
The institute focuses on research and education in data science, machine learning, and artificial intelligence across diverse fields, including neuroscience, precision medicine, climate resilience, sustainability, public-sector innovation, and social sciences.
Collaborate to develop data science and AI, and accelerate breakthroughs.