Engineering a dashboard and API for AI tools—Natural Language Processing and Machine Learning—to search and discover how datasets are referenced by authors in scientific texts
Julia Lane (NYU Wagner Graduate School of Public Service) (pictured)
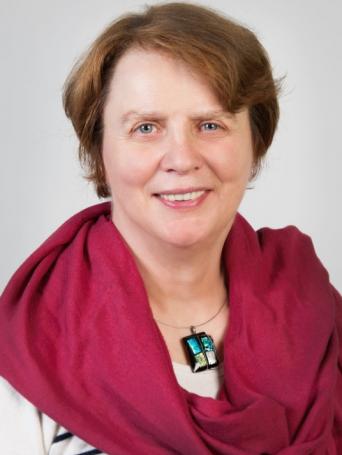
Democratizing Data
PI Julia Lane (NYU Wagner Graduate School of Public Service)
The call for better data and evidence for decision-making has become very real in the US as evidenced by the passage of both the Foundations of Evidence-based Policymaking Act (Evidence Act) and the CHIPS+ Act, establishing a National Secure Data Service. The challenge to be addressed is finding out not just what data are produced but how they are used – in essence, to build an Amazon.com for data -so that both governments and researchers can quickly find the data and evidence they need.
JHU is participating in a massive effort that started five years ago which has been focused on finding out how data are being used, to answer what questions, and find out who are the experts, by mining text documents that are hidden in plain sight – in the text of scientific publications, government reports and public documents. The core idea has been to use Artificial Intelligence tools – Natural Language Processing and Machine Learning – to search and discover how datasets are referenced by authors in scientific text. A major Kaggle competition, a follow on conference, and a piece in the Harvard Data Science Review, showed not only that it was possible to find data, but just as with Amazon, when it is possible to see how datasets are used the results are enormously powerful.
The current work—which is sponsored by agencies such as NSF’s National Center for Science and Engineering Statistics (NCSES), NSF’s Technology, Innovation, and Partnerships (TIP) Directorate, the Department of Education’s National Center for Education Statistics (NCES) and the US Department of Agriculture, and private foundations such as Schmidt Futures and the McGovern Foundation—has generated a prototype API and a dashboard that can be used – so that, for example, agencies can document dataset use for Congress and the public, program managers can identify investment opportunities rapidly and researchers can more easily build on existing knowledge rather than redoing things from scratch. JHU is building out both a validation server as well as researcher access through its SciServer platform. We expect the results to make a huge difference in understanding the public good produced by government dataset – to paraphrase Lee Platt’s aphorism about HP – “If government knew what government knows, it would be three times more productive”.