Using deep learning to predict human-compatible optogenetic proteins could dramatically accelerate early drug discovery and protein engineering outcome.
Dr. Sapna Sinha (Schmidt Fellow)(pictured)
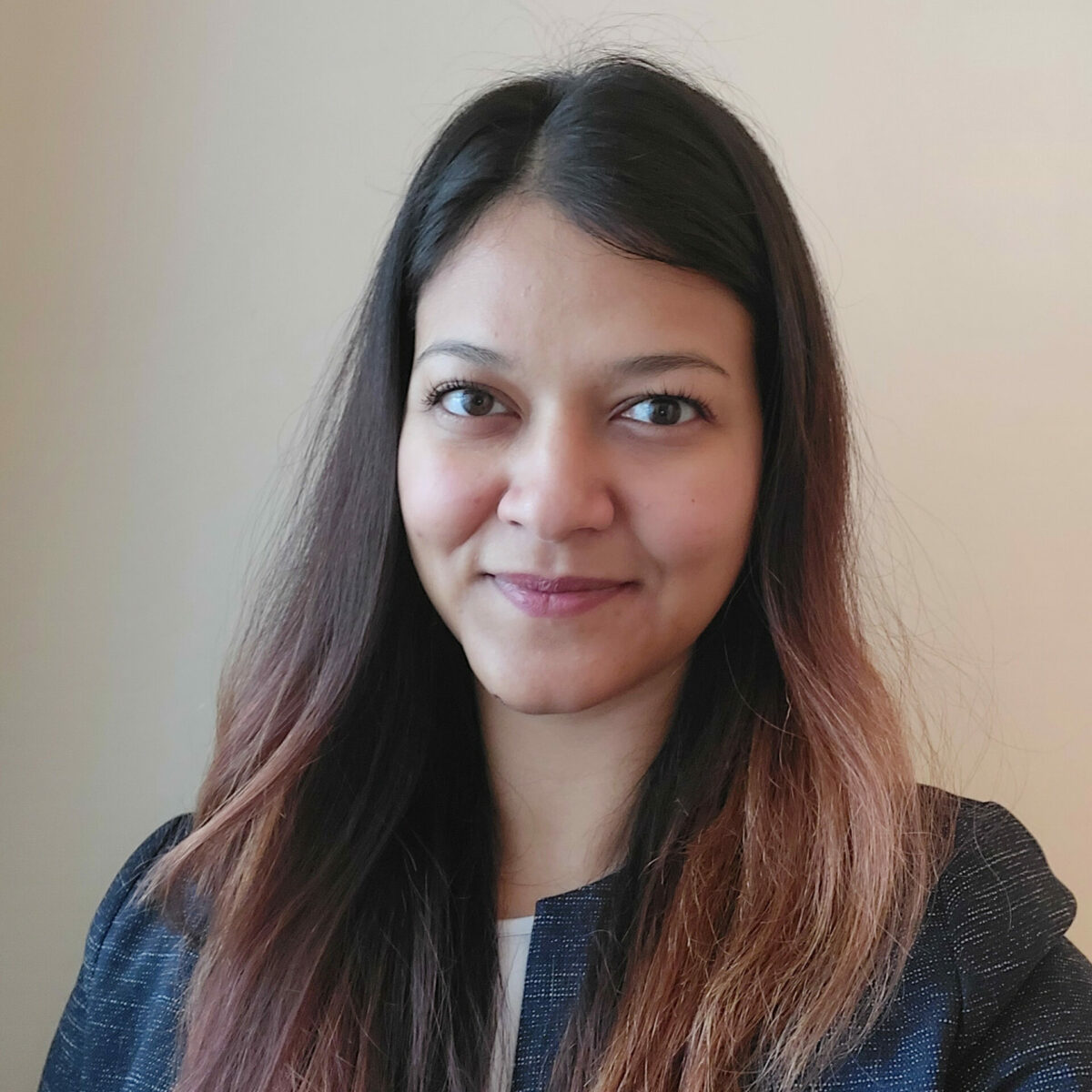
Humanizing Optogenetics
PI Dr. Sapna Sinha (Schmidt Fellow, MIT)
Recent breakthroughs in deep learning models applied to protein sequences and structural data have resulted in the ability to synthesize realistic human-like protein sequences de-novo (ProtGPT, ProtBERT), to predict protein structure from a sequence (AlphaFold, ESM-2) and to predict protein sequences that can fold into physically plausible 3D configurations with high accuracy (AlphaFold, ESM, ProteinMPNN). Sequence information of a protein can be used to predict binding to major histocompatibility complex (MHC) molecules (NetMHCPan-4.0) which is used as an indicator of immunogenicity. Humanizing Optogenetics, an initiative of Sapna Sinha of MIT (PI), proposes distributed computational pipelines based on these models for automated, high-throughput, in-silico candidate generation for engineering human-compatible optogenetic proteins that can form the basis of new devices for the optical neural control of prosthetics.
In this vision, deep learning models seeded with optogenetic protein structures are employed to sample large quantities of candidate sequences with potential for optogenetic function. Low immunogenic candidates are then computationally folded into likely 3D configurations, refined and evaluated for similarity against known optogenetic libraries. Top scoring in-silico results are then passed along to the laboratory where function is assessed in-vitro and, if confirmed, optimized by traditional protein engineering methods.
With sufficient ease of use, flexibility and computational scaling, such screening pipelines have the potential to systematically and efficiently explore vast regions of target sequence space in search of suitable candidates. If even only a fraction of deep learning-generated candidates can fold into the desired structure and exhibit the desired function in-vitro, this approach could dramatically accelerate early drug discovery and protein engineering outcomes. Framework configurability allows the basic steps to be extended with additional or alternative processing to optimize the yield of candidates found to be compatible and functional. Furthermore, a successful framework incorporating these models is a general-purpose tool, and will be of interest in many protein engineering and gene therapy applications beyond Optogenetics.